Hype vs. substance
I’m coining disruptobloat to describe a distinct season that any major technology goes through:
VC funds are running high for 50 versions of the same use case
everyone’s LinkedIn tagline indicates they’ve been a decade-old expert in [some new tech]1,
headlines slowly move from “all companies are building in [tech]” to “are consumers fed up with [tech]?” to the merciless butt-of-the-joke and “what went wrong” .
Disruptobloat is a phenomenon of overproduction: [some new tech]-driven products flood the market, diluting the perception of value in the short term.
It’s a race towards the same thing: discovering a sticky use case that shapes new customer behaviors and accrues value. It’s not a bug; it’s a necessary step in the evolution, and a good thing! The bigger the disruptobloat, the faster we get to breakthroughs, because we iterate through ideas faster.
from breaks it down this way:Level 1: Do what we do cheaper: (…) automate routine tasks.
Level 2: Do what we do, just do it better: (…) opportunities for qualitative improvements. A major investment bank, for instance, recently used AI to automate much of its unit test coverage. This reduced costs and allowed for more comprehensive testing, improving overall software quality.
Level 3: Do entirely new things. This is where the true potential of AI begins to show (…) But here’s the rub: most businesses are stuck at Level 1 or Level 2. They’re using AI to shave costs or incrementally improve processes, missing the opportunity to strategically rethink what their business could look like (…)
The things is, everyone is trying to “strategically rethink what their business could look like”, but it’s tough. We’re all conditioned to think through the implicit constraints of our day-to-day life, and rethinking happens when we ignore those constraints. For existing businesses they’re also the constraints of the ossified ecosystems of customers, partners, revenue, and profit.
The Commoditization of AI Models
There’s a saying that originated during the Gold Rush: "When people dig for gold, sell shovels”, used often to describe a business strategy: instead of directly participating in a competitive and speculative market, provide the essential tools and services for that market. The problem with shovels, though, is that they’re fungible, and turns out that AI models are too.
Let’s assume that no provider releases a model that is orders of magnitude better than the competition for a long enough time for it to strategically matter2. Where does the value accrue, then? In other words, what kinds of products will be able to build a moat?
The application layer3 - the surfaces, apps, sites through which users will interact are:
most likely to shape new behaviors, teaching users to do entirely new things, so
likely to accrue much more value over time by building new markets
No surprise, then, that it’s worth to compete with hundreds (if not thousands) of startups for the same use cases. 75% of the last YC batch were AI startups - and that’s just one venture fund!
This quote from a16z gives a snapshot idea of where the effort is going; a progression from lowest hanging fruit to doing new things:
AI tools that run on top of existing software (think: automatic meeting notes for Zoom meetings)
AI tools that run on top of existing software that have a shot of displacing that existing software (think: meeting notes for Zoom Meetings…where said company then builds video conferencing and pitches you to ditch Zoom)
AI tools that turn into labor — a net-new category, completely untouched by software until this point (think: the software conducts the meeting for you!)
Hence, disruptobloat.
The Unbundling of GPTs
This race between existing companies and 0→1 startups is a pure Product Discovery Challenge. In theory, model providers should have an advantage, a result of having collected 2 years of usage data. Looking for insight from OpenAI’s marketplace of GPTs returns pretty boring data; I’m sure the actual conversations are more illuminating, but probably not a slam dunk. GPTs show that people are using LLMs for things they know they can use LLMs for. The breakthrough comes when a product, and a team behind it, figures out how to teach people to do entirely new things.
It's reminiscent the unbundling of Craigslist - just as its various boards were split into specialized services, many of them reaching unicorn status at some point, we'll see the same happening - and much faster - to GPTs, with each product trying to solve a specific problem better than a one-size-fits-all chat window.
Vertical Integrator Strategy
Last week,
published Vertical Integrators (which seeded a lot of thinking behind this post). In the context of AI's disruptobloat and the commoditization of models, the vertical integrator strategy becomes particularly relevant: it’s a way of building a moat, and it’s where incumbents have an advantage. From :Vertical Integrators are companies that:
Integrate multiple cutting-edge-but-proven technologies.
Develop significant in-house capabilities across their stack.
Modularize commoditized components while controlling overall system integration.
Compete directly with incumbents.
Offer products that are better, faster, or cheaper (often all three).
NVIDIA is an example of this strategy on steroids, building ecosystems around the core technologies to control the entire technology stack, especially as base models become commoditized:
Hardware (GPUs, A100, H100, DGX, Jetson)
Software (CUDA, TensorRT)
Platforms: NVIDIA Omniverse for 3D simulations, NVIDIA Clara for healthcare
Robotics Lab and Issac Sim robot simulator
NVIDIA DRIVE for autonomous vehicles, both hardware and software (DRIVE AGX, DRIVE OS).
Not all incumbents are or will be competing in every layer now, but the point is that they have the capability to do so, whether by building or acquisitions. As a16z explains, using Stripe and Square as an example for fintech-adjacent services:
“This is the flaw with looking at Square and Stripe and calling them commodity players. They have the distribution. They have the engineering talent. They can build their own TiVo. It doesn’t mean they will, but their success hinges on their own product and engineering prowess, not on an improbable deal with an oligopoly or utility. ”
The Parting Gift
One of the early goals I had for this post was to pinpoint the killer use cases, which, in retrospect, is a tall order for a few hours of research. Still, as the hype slows down, there are a few corners of disruptobloat that I’m paying attention to:
Lowering the cost of previously highly desired, but high-price services - legal, finance, healthcare - where low cost can create massive demand. From a16z (again): “LVMH likely spends tens of millions of dollars a year fighting counterfeit goods, sending cease and desist letters, cooperating with law enforcement, etc. How many small Shopify merchants might want the exact same service? All of them! How many could spend $50M/year? None of them. How many might spend $1,000/year? Maybe all of them?”
Democratizing complex skills, like we did with coding. Most of the narrative around the LLM-assisted programming focuses on cost savings, but the magic of it is that it enables people to entirely new things they couldn’t do before. We’ve heard this for a while, first with coding bootcamps, then with no-code apps, but those came with limitations. Now there are none.
Hyper-personalization at scale, across any consumer activity
AI + Robotics
Climate tech4
The killer use case is somewhere out there, unrefined and drowning in noise. Whether - or when - the market will be ready is another question.
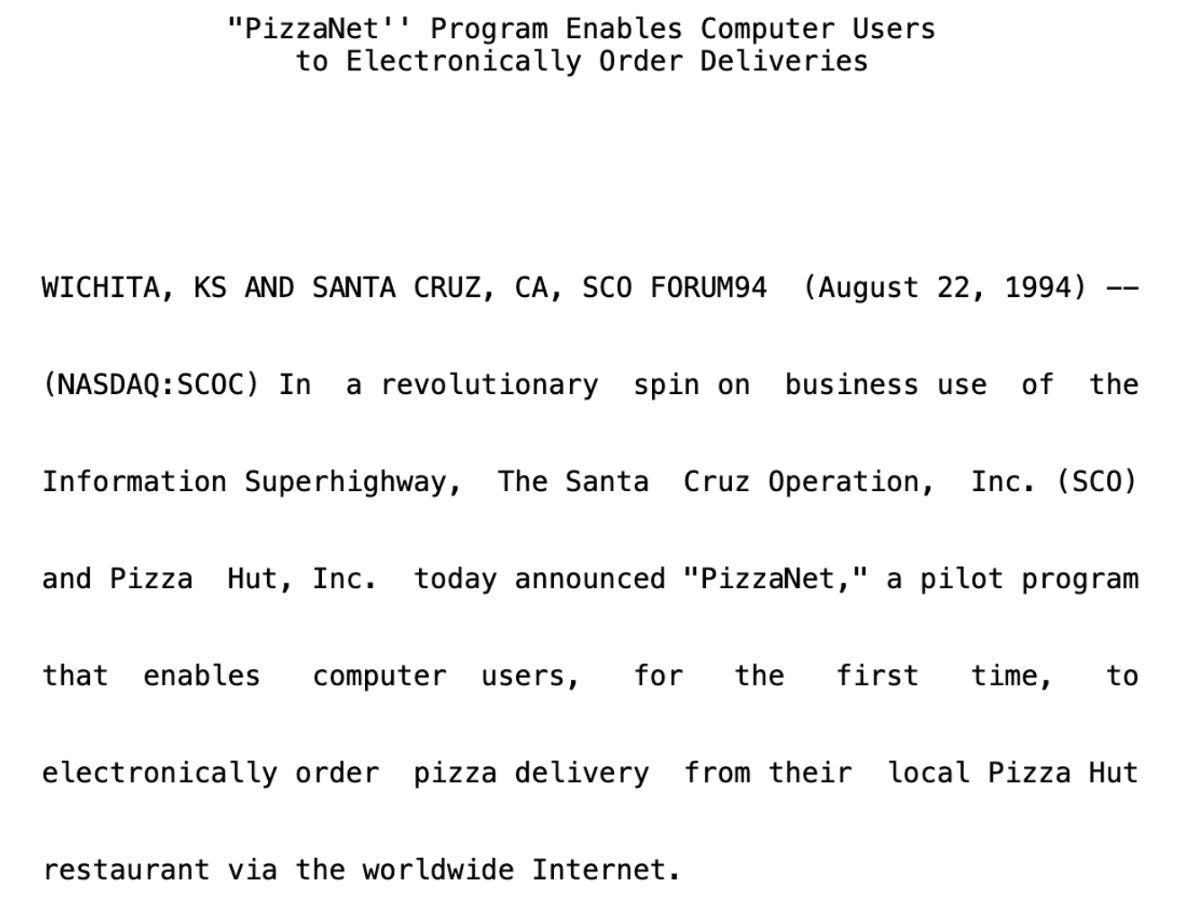
PS. Thank you Claude for brainstorming and editing.
I say this shamelessly, as my own LinkedIn includes the trifecta of crypto, AI, and Labs
I’m going against the grain on the narrative around GPT-NEXT: even if they get the “first mover” advantage on the next gen model, the competition is unlikely to be far behind. If for no other reason, then just because the people building the models move from one company to another.
I’m skipping past the infrastructure layer, because it’s table stakes
YC’s Request for Startups is a great inspiration for “AI is the means, not the end” product thinking.
Thanks for the shout out. It's always the incumbents challenge. On the one hand you could argue it requires imagination -- and the means leadership.
On the other hand you could argue that managers should focus on 1 and 2 and let new entrants do 3. Shareholders can always move their capital when the incumbent moves into steady-state and then decline.